Data protection regulators publish myth-busting guidance on machine learning
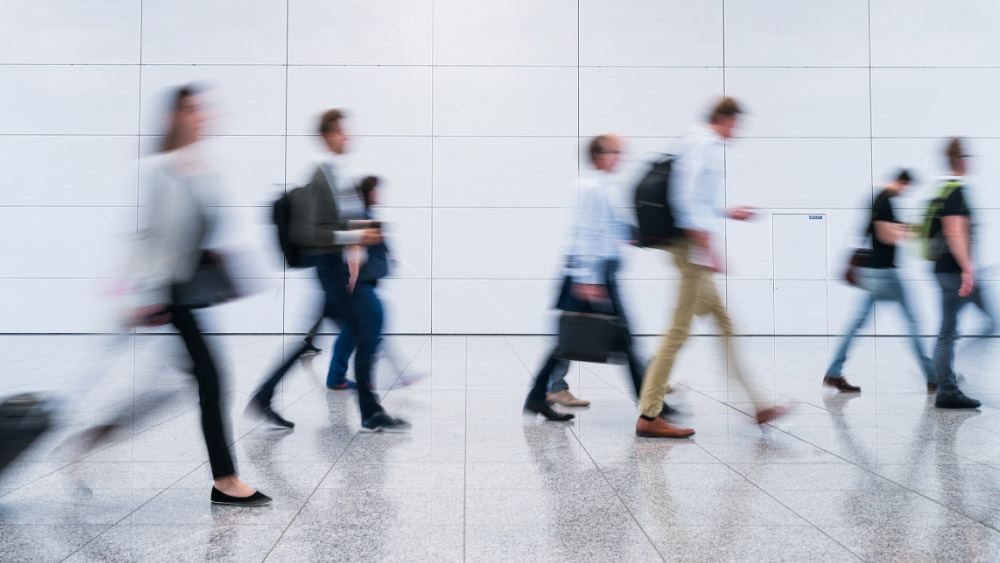
In its proposed AI Regulation (“AI Act”), the EU recognises AI as one of the most important technologies of the 21st century. It is often forgotten, however, that AI is not one specific type of technology. Instead, it is an umbrella term for a range of technologies capable of imitating certain aspects of human intelligence and decision-making – ranging from basic document processing software through to advanced learning algorithms.
One branch of the AI family is machine learning (“ML”), which uses models trained by datasets to resolve an array of complicated problems. The specific form and function of an ML system depends on the tasks it is intended to complete. For example, the ML system could be used to determine likely trends of categories of persons to default on loan agreements through the processing of financial default information. During the development and training of their algorithms, ML systems begin to adapt and recognise patterns within its data. They can then use this training to interpret new data and form outputs based on the intended process.
The use of ML systems gives rise to several questions which lawyers and compliance professionals may be uncertain about answering. For example: How is the data interpreted? How can an outcome be verified as accurate? Does the use of large datasets remove any chance of bias in my decision making?
In attempt to resolve some of these issues, the Agencia Española de Protección de Datos (Spain’s data regulator) (“AEPD”) and the European Data Protection Supervisor (“EDPS”) have jointly published a report addressing a number of notable misunderstandings about ML systems. The joint report forms part of a growing trend among European data protection regulators to openly grapple with AI issues, recognising the inextricable link between AI systems – which are inherently creatures of data – and data protection law.
In this article we elaborate on some of these clarifications in the context of a world of developing AI and data privacy frameworks.
Causality requires more than finding correlations
As a brief reminder to ourselves, causality is “the relationship that exists between cause and effect” whereas correlation is “the relationship between two factors that occur or evolve with some synchronisation”.
ML systems are very good at distinguishing correlations with datasets but typically lack the ability to accurately infer a causal relationship between data and outcomes. The example given in the report is that, given certain data, a system could reach the conclusion that tall people are smarter than shorter people simply by finding a correlation between height and IQ scores. As we are all aware, correlation does not imply causation (despite the spurious inferences from data that can be made, for example from the correlation between the per capita consumption of mozzarella cheese and the number of civil engineering doctorates awarded).
It is therefore necessary to ensure data is suitably vetted throughout the initial training period, and at the point of output, to ensure that the learning process within the ML system has not resulted in it attributing certain outcomes with correlating, but non-causal, information. Having some form of human supervision to determine when certain variables are being overweighted within the decision process may assist in doing so and allow intervention when bias is detected at an early stage in processing.
Training datasets must meet accuracy and representativeness thresholds
Contrary to belief, a greater variety in data does not necessarily mean that it is a better dataset or better able to mitigate bias. It is instead better to have a focused dataset that accurately reflects the trend being investigated. For example, having data on all types of currency in relation to their conversion to dollars is not helpful when seeking to find patterns and trends in the fluctuation in conversion between dollars and pounds sterling.
Furthermore, the addition of too much of certain data may lead to inaccuracies and bias in outcomes. For example, as noted in the report, the use of light-skinned male images in a dataset used to train facial recognition software will be largely unhelpful in correcting any existing biases for ethnicity or gender within the system.
The General Data Protection Regulation (“GDPR”) requires that processing of personal data be proportionate to its purpose. Care should therefore be taken when seeking to increase the amount of data in a dataset. Substantial increases in data used to produce a minimal correction in a training dataset, for example, may not be deemed proportionate and lead to breach of the requirements of the Regulation.
Well-performing machine learning systems require datasets above a certain quality threshold
It is equally not necessary that training datasets be completely error-free. Often, this is not possible or commercially feasible. Instead, datasets should be held to a certain quality that allows for a comprehensive and sufficiently accurate description of data. Providing that the average result is accurate to the overall trend, ML systems are typically able to deal with a low level of inaccuracy.
As the report notes, some models are even created and trained using synthetic data (artificially generated datasets – described in greater detail in our earlier article on the subject) that replicate the outcome of real data. In some cases, synthetic data may even be aggregated data from actual datasets which retains the benefit of accurate data trends while removing many compliance issues associated with personally identifiable information.
This is not to say that organisations should not strive to attain an accurate data set and, in fact, under the AI Act it is a mandatory requirement that a system’s data be accurate and robust. Ensuring accuracy and, where relevant, currency of personal data is also a requirement under the GDPR. However, it is important to remember that ‘accuracy’ in the context of ML need not be an absolute value.
Federated and distributed learning allows the development of systems without sharing training data sets
One approach proposed for the developing of accurate ML systems, in the absence of synthetic data, is the creation of large data-sharing repositories, often held in substantial cloud computing infrastructure. Under another limb of the EU’s digital strategy – the Data Governance Act – the Commission is attempting to promote data sharing frameworks through trusted and certified ‘data intermediation services’. Such services may have a role to play in supporting ML. The report highlights that while this means of centralised learning is an effective way of collating large quantities of data, this method comes with its own challenges.
For example, in instances of personal data, the controller and processor of the data must consider the data in the context of their obligations under the GDPR and other data protection regulations. Requirements regarding purpose limitation, accountability, and international transfers may all therefore become applicable. Furthermore, the collation of sensitive data increases the interest of other parties, particularly those with malevolent intent, in gaining access. Without suitable protections put in place, a centralised dataset with large quantities of data may become a honeypot for hackers and corporate parties seeking to gain an upper hand.
The report offers, as an alternative, the use of distributed on-site and federated learning. Distributed on-site learning involves the data controller downloading a generic or pre-trained model to a local server. The server then uses its own dataset to train and improve the downloaded model. After this is completed, there is no further need for the generic model. By comparison, with federalised learning the controller trains a model with its own data and then sends only its parameters to a central server for aggregation. It should be noted however, that often this is not the most efficient method and may even be a barrier to entry or development for smaller organisations in the ML sector, due to cost and expertise restrictions.
Once deployed, machine learning models performance may deteriorate until further trained
Unlike other technologies, ML models are not plug in and forget systems. The nature of ML is that the system adapts and evolves over time. Consequently, once deployed, an ML system must be consistently tested to ensure it remains capable of solving the problems for which it was created. Once mature, a model may no longer provide accurate results if it does not evolve with its subject matter. For example, a ML model aimed at predicting futures prices of coffee beans will deteriorate if it is not fed new and refreshed data.
The result of this, should the data not be updated for some time, is an inaccurate model that will produce tainted, biased, or completely incorrect judgements and outcomes (a situation known as data drift). This may also occur in instances where the interpretation of the data changes within the algorithm while the general distribution does not (known as concept drift). As the report notes, it is therefore necessary to monitor the ML system to detect any deterioration in the model and act on its decay.
A well-designed machine learning model can produce decisions understandable to relevant stakeholders
Perhaps the fault of popular media, there is a recurring belief that the automatic decisions taken by ML algorithms cannot be explained. While this may be the case for a select few models, a well-designed model will typically produce decisions that can be readily understood by stakeholders.
Some of the factors which are important in terms of explainability are understanding which parameters were considered and their weighting in decision making. The degree of ‘explainability’ demanded from a model is likely to vary based on the data involved and the likelihood of a decision to impact the lives of data subjects (if any). For example, far greater explainability would be expected from a model that deals with credit scoring or employment applications than those tasked with predicting futures markets.
It is possible to provide meaningful transparency to users without harming IP rights
A push towards transparency and explainability has naturally led many to question how to effectively protect trade secrets and IP when everyone can see how their models and ML systems behave. As the report highlights, transparency and the protection of IP are not incompatible, and there are several methods of providing transparency to users without harming proprietary know-how or IP. While users should be provided with sufficient information to know what their data (particularly personal data) is being used for, this does not necessarily mean that specific technical details need disclosed.
The report compares the requirement to the provision of advisory leaflets with medicine. It is necessary to alert users to what may happen when using the medicine (or model/system) without providing an explanation of how this is specifically achieved. In cases of personal information, further explanation may be required to comply with the principles set out in applicable data protection regulation. At a minimum, data processors and controllers should properly inform users and subjects of the impacts of the ML and its decision-making on their daily lives.
Further protections for individuals may be achieved through certification in accordance with international standards, overt limitations of system behaviour, or the use of human moderators with appropriate technical knowledge.
Machine learning systems are subjects to different types of biases
It is often assumed that bias is an inherently human thing. While it is correct to say that a ML system is not in itself biased, the system will perform as it is taught. This means that while ML systems can be free from human bias in many cases, this is entirely subject to its inherent and learned characteristics. Where training or subsequent data is heavily one-sided or too much weight is ascribed to certain data points, the model may interpret this ‘incorrectly’ therefore leading to ‘biased’ results.
The inherent lack of ‘humanity’ in these systems does however have its drawback. As the report notes, ML systems have a limited ability to adapt to soft-contextual changes and unforeseen circumstances, such as changes in market trends due to new legislation or social norms. This point further highlights the need for appropriate human oversight of the functioning of ML systems.
Predictions are only accurate when future events reproduce past trends
‘ML systems are capable of predicting the future’ is perhaps one of the most common misconceptions with the technology. Rather, they can only predict possible future outcomes to the extent that they reflect the trends of previous data. The likelihood that you buy coffee on a Monday morning when you have habitually done so since starting your job indicates that it is certainly likely that you will do so this coming Monday, but it does not guarantee that you will do so, or that an unforeseen event will prevent you from doing so.
Applying this to the context of commerce, a ML system may be able to (with relative accuracy) predict the long-term trend of a particular futures market but cannot guarantee with absolute certainty that market behaviour will follow suit, particularly in the case of black swan events, such as droughts or unexpected political decisions.
To increase the chances of a more accurate outcome, organisations should seek to obtain as large a data set as possible, with as many variables considered as obtainable, while maintaining factual accuracy to the trends of data they utilise. This will allow the ML system to better predict behavioural responses to certain data and therefore produce more accurate prediction outcomes.
A system’s ability to find non-evident correlations in data can end up with the discovery of new data, unknown to the data subject
A simultaneous advantage and risk of ML systems is their capacity to map data points and establish correlations previously unanticipated by the system’s human designers. In short, it is therefore not always possible to anticipate the outcomes they may produce. Consequently, systems may identify trends in data that were not previously sought, such as predispositions to diseases. While this may be beneficial in certain circumstances, such as health, it may equally be unnecessary or inappropriate in other contexts.
Where these ML systems begin processing personal data beyond the scope of their original purpose, considerations of lawfulness, transparency, and purpose limitation under the GDPR will be engaged. Failure to appropriately justify the processing of personal data in this manner without clear purpose may result in breach of the Regulation and the subsequent penalties that accompany it.
Get in touch
For more information on AI and the emerging legal and regulatory standards visit DLA Piper’s focus page on AI.
You can find a more detailed guide on the AI Regulation and what’s in store for AI in Europe in DLA Piper’s AI Regulation Handbook.
To assess your organisation’s maturity on its AI journey in (and check where you stand against sector peers) you can use DLA Piper’s AI Scorebox tool.
You can find more on AI, technology, data privacy, and the law at Technology’s Legal Edge, DLA Piper’s tech-sector blog and Privacy Matters, DLA Piper’s Global Privacy and Data Protection resource.
DLA Piper continues to monitor updates and developments of AI and its impacts on industry in the UK and abroad. For further information or if you have any questions, please contact the authors or your usual DLA Piper contact.