Emerging definitions: Capturing the essence of Artificial Intelligence
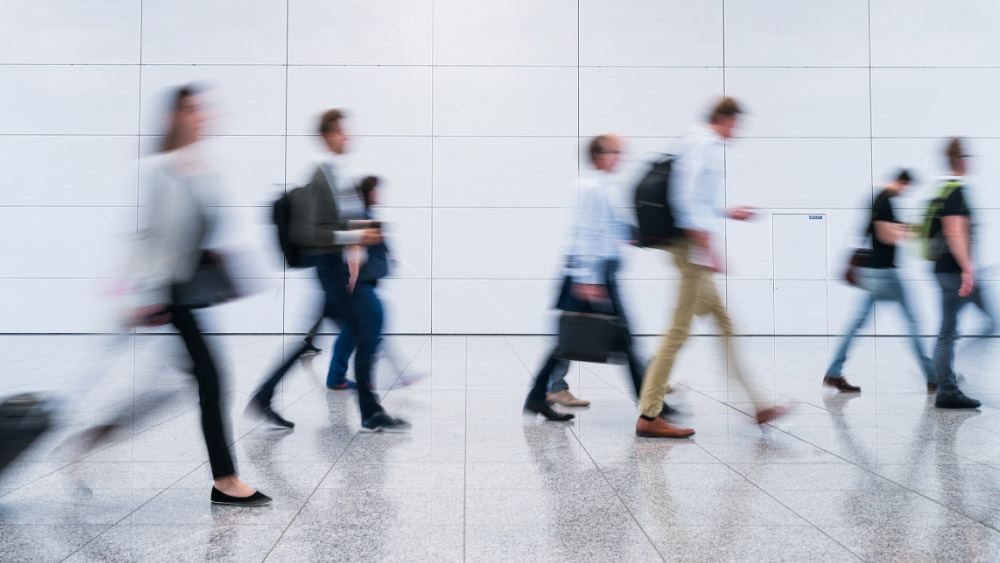
Glimpsing blue whales in your tears
What’s the difference between a teardrop and an ocean? The question might seem ridiculous, but oceans and teardrops have more in common than might first seem the be the case. Both are largely composed of salty water, both have creatures living in them (tiny micro-organisms in the case of teardrops), the average temperature of a teardrop and an ocean are comfortably within the range where water is liquid. The real difference is volume – but add 1024 (for the non-mathematically inclined, that’s a one with twenty-four zeroes after it, or 1,000,000,000,000,000,000,000,000) teardrops together, and you have all the oceans on Earth.
This illustrates a truth about emergent properties. At certain point, more ceases to be just more of the same, and becomes something qualitatively different. Properties emerge that were not obvious in the smaller collections. One teardrop is scarcely noticeable. A few hundred is a wet handkerchief at the end of a ‘weepy’ movie. Tides, dramatic coastlines, waves you can surf on and habitats that can support everything from Arctic penguins to tropical corals emerge as you move up the size scale from a teardrop to an ocean.
Bits, bytes and yottabytes
This same phenomenon of emergent properties applies in computer science as well. The journey from the room-sized earliest valve computers of the 1940s to today’s fully connected world illustrates this. Today, everyone carries a networked supercomputer in their pocket – which then gets used for critical tasks such as checking social media or booking food delivery. Few of these activities would be obvious to anyone considering the Colossus in Bletchley Park in 1944, and the modern connected world is certainly an emergent property that would not be obvious going from one transistor to billions.
Many of the principles that underpin today’s cutting-edge AI systems have their roots at the very dawn of the electronic computing revolution in the ‘40s and ‘50s. Whilst we might think of neural network based systems as being the current state-of-the-art in machine learning, the origins of today’s systems can be traced back to the perceptron, developed in the late 1950s. That device demonstrated the uncanny effects emerging from a few simple rules, and with an input of only 20 x 20 photodiodes, it could be trained to recognise simple image sets – rectangle vs. oval for example.
If those first perceptrons had a mere 400 ‘trainable’ parameters(20 x 20), today’s state-of-the-art natural language processing systems might have 175 billion or more parameters – nine orders of magnitude greater. At their heart, the individual artificial ‘neurons’ that are used in neural networks are simple – a few inputs connected to the neuron. Each input’s connection is assigned a weight. In mathematical terms the input value is multiplied by the weight. The neuron then sums all of the results of the multiplications, and the result determines the neuron’s activation level (often after the result has been normalised to fit within a particular activation range).
In isolation, a single neuron is entirely predictable and comprehensible. There are no obvious difficulties with bias or ethics. None of the various potential mischiefs that are addressed in various white papers and draft regulations on AI manifest themselves.
It is the emergent properties arising from scaling from one neuron to hundreds of billions that create the potential for these effects.
A question of definition
Lawyers love a definition. They provide the illusion of certainty, despite almost every word having multiple nuanced meanings. Present two litigators with the same definition in a case that turns on its meaning, and even the most seemingly crystal-clear drafting will crumble into ambiguity and counterarguments. Despite this, definitions in both legislation and contracts are critically important, and will delineate the scope of obligations, or the landscape of proscribed behaviours.
In the field of AI, commentators will often spend time examining the apparent impossibility of defining ‘intelligence’, and therefore the double impossibility of defining AI by reference to intelligence.
Those grappling with definitions in contracts or legislation will often take either a technology-based approach, a purposive approach, or straddle the two.
A technology-based approach might provide the advantage of a very clear test as to whether a particular system is or is not caught by the definition. For example, “AI means any system using a neural network” might provide relative clarity as to which systems are caught, but it is weighed down with obvious disadvantages. What happens when technology moves on? What about systems that exhibit the mischiefs that you want to regulate, but which might not rely on the relevant technology? What about systems which might use neural networks in a simple or harmless way, and upon which you don’t intend to impose the obligations that turn on the definition?
Purposive approaches tend to focus on the use case for a system, often by reference to skills formerly reserved to human beings, or by reference to a system’s capacity to “learn”.
The former (replacement of humans) presupposes that the replacement of a human is either absolute or particularly relevant. In many cases relatively simple technologies used on combination can allow one employee to undertake the work previously carried out by several. Particularly when tasks are restructured to include a ‘self-service’ element, any definition that turns on machines doing tasks previously reserved to humans risks capturing technologies as banal as ATMs or self-checkout systems in supermarkets. These systems might have replaced bank tellers and checkout clerks respectively, but few of us would consider them AIs worthy of special control or regulation.
The latter (capacity to learn) exposes a fallacy in popular thinking about AI systems. Whilst the systems might learn during a training phase, those deployed in production environments tend to be in a fixed state for inference, with new versions from additional training only being rolled out (and then in a similarly fixed state) periodically. Any systems deployed for inference would not themselves have the capacity to learn, and therefore would be missed by a definition that focussed purely on that quality.
Exactly these challenges are present in the definition of AI System in the latest draft of the EU AI Regulation at the date of writing. The updated version in the Compromise Text published by the European Council in November 2021 reads:
‘artificial intelligence system’ (AI system) means a system that
- receives machine and/or human-based data and inputs,
- infers how to achieve a given set of human-defined objectives using learning, reasoning or modelling implemented with the techniques and approaches listed in Annex I, and
- generates outputs in the form of content (generative AI systems), predictions, recommendations or decisions, which influence the environments it interacts with;
The content of Annex 1 listing the techniques and approaches remains as per the original draft:
(a) Machine learning approaches, including supervised, unsupervised and reinforcement learning, using a wide variety of methods including deep learning;
(b) Logic- and knowledge-based approaches, including knowledge representation, inductive (logic) programming, knowledge bases, inference and deductive engines, (symbolic) reasoning and expert systems;
(c) Statistical approaches, Bayesian estimation, search and optimization methods.
That definition’s reference to the listed technologies or techniques in Annex 1 casts the net wide in order to attempt to future-proof the definition. This will result in a very broad class of systems being considered ‘AI Systems’, which in turn would capture operators of many existing systems which do not and cannot exhibit any of the potentially problematic emergent properties the regulation is primarily designed to control. As a result, (and assuming that something similar to this definition remains in the version of the regulation that is enacted), a regulatory burden and the attendant costs of compliance will fall upon a far wider range of systems and operators than is strictly necessary.
An emerging alternative
Ideally, any definition of AI should be reasonably clear, technology agnostic, and capture those systems which might exhibit the relevant mischiefs whilst not throwing the net too widely and imposing obligations upon those which pose no risk.
The authors propose that definitions should focus on the emergent properties of complexity, and the unexpected behaviours that might result, rather than any particular technology or purpose.
Consider the following as an example:
An “AI System” is any automated data processing or decision making system:
- which is sufficiently complex that the whole exhibits emergent properties not present in the components that make up the system; and
- where those emergent properties could manifest as behaviours that the operator or user of the system might not reasonably predict.
This definition focuses on the idea that the mischiefs primarily to be controlled via any obligations attaching to AI systems are those arising unexpectedly.
Any operator using a system to deliberately carry out activity that is biased, discriminatory, untrustworthy or fraudulent would bear liabilities at law already for such prejudiced or nefarious conduct, whether or not it involved any AI system. The nuance with AI systems is that such behaviours might not be intended, but (if the system is not well designed and monitored) might manifest nevertheless.
Applying this definition, an operator who deliberately set up a system to be discriminatory might not be caught by this definition, but would be caught by existing legislation controlling the relevant behaviours. To the extent that any relevant offences required guilty intent (mens rea for those who prefer the Latin), such intent would clearly be present in such a case.
However, the operator who set up a system which exhibited unexpected biases or which occasionally and unpredictably produced wrong results, would be caught by this new definition. Therefore any controls applied in attendant legislation using this definition would catch those systems that might benefit from those controls. Examples of relevant controls upon operators might include:
- design systems to minimise the possibility that such behaviours might manifest;
- ensure that the operator checks regularly that problematic behaviours are not present; and
- have clear appeals processes to human decision makers for those persons who might be affected on the occasions that such behaviours do manifest.
For those who prefer a belt-and-braces copper-bottomed approach, the definition could be extended with a third limb to specifically include systems where the problematic behaviours have been deliberately manifested in the system, so that it would not be necessary to rely entirely on current anti-discrimination laws etc. to control those bad actors using complex systems for antisocial purposes.
Intelligent regulation
While imagining possible alternative definitions provides a diverting philosophical challenge, the definitions that AI operators need to have in mind are those that eventually emerge in relevant legislation.
From an EU perspective, it seems likely that the EU AI Regulation will retain a definition reasonably close to the one in the current drafts. Just as the GDPR’s definition of ‘personal data’ became a de facto standard, the definition of AI system in the EU AI Regulation may therefore become a benchmark definition within the industry. Other competing (and potentially incompatible) definitions are likely to be contained in laws regulating aspects of AI deployment in the US, UK, China and elsewhere. In the US, and possibly the UK, legislators might apply a more sectoral approach, targeting specific AI uses in particular industries. This sectoral approach may result in more of a patchwork of AI definitions dependent upon context.
Against that backdrop, it will be for the regulators, as the enforcers of these new rules, to apply their own philosophy to the legislative definitions. Again, we can look to experiences in the realm of data protection to see how it might play out, although we need to go back further than GDPR. In the early years after the 1995 Data Protection Directive was enacted into the laws of Member States we saw the then brand new definition of personal data tested time and again – What constituted personal data? How easily identifiable did a data subject need to be? Was it really forbidden for parents to video school nativity plays? In deciding these questions, the regulators were bound to follow the text of the definition, but in doing so inevitably revealed their own perspective and philosophy.
The same will be true of the key definitions of AI in these new laws – although given the breadth of the definitions, the regulators will enjoy even more leeway in interpreting the limits of the definitions in accordance with their own concepts and purposes. With that in mind, if those regulators pay heed to whether particular systems do or don’t exhibit potentially problematic emergent properties, that could provide useful direction to where enforcement efforts ought to be concentrated.
Next Steps
For those looking to take advantage of the promise offered by state-of-the-art AI systems within their organisation, any definition proposed by legislators (whether the broad definition likely to be adopted in the EU, or potentially more targeted definitions that might find favour elsewhere) is likely to apply to that system one way or another. Focussing on ensuring the potential emergent properties (and the negative effects that might arise from them) is critical. Comments from governments and proposed regulatory regimes have focussed on explainability, transparency, trustworthiness and the ability to spot and eradicate biases that might emerge. Designing systems with these goals in mind is the best way to ensure that a ‘compliance by design’ approach is adopted as regulations evolve.
Of course business does not stand still and wait for regulations to be settled, implemented and understood. As such there will be a myriad of complex technology deployment activities going on right now around the globe. The concern therefore is that emerging regulation might cut-across a business need, a product roadmap or a new line of business. To solve that concern, organisations must take account of the potential new regulations before they arrive so as to ensure that they have a defensible, auditable, and ultimately reasonable deployment approach (in the context of their sector).
For more information on AI and the emerging legal and regulatory standards, contact Gareth Stokes, Imran Syed, Mark O’Conor or your usual DLA Piper contact. To assess your organisation’s maturity on its AI journey in (and check where you stand against sector peers) you can use DLA Piper’s AI Scorebox tool, available at https://aiscorebox.dlapiper.com/.
You can find more on AI, technology and the law at Technology’s Legal Edge, DLA Piper’s tech sector blog: https://www.technologyslegaledge.com/.